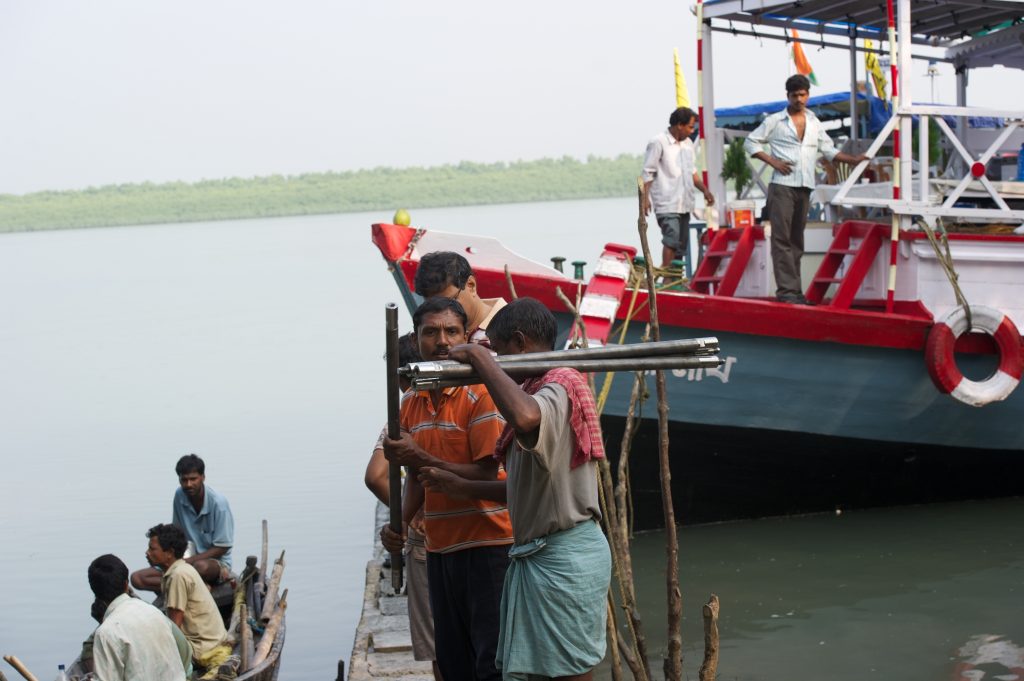
February 14, 2013
“Mark but this flea, and mark in this, How little that which thou deniest me is; It suck’d me first, and now sucks thee, And in this flea our two bloods mingled be. Thou know’st that this cannot be said A sin, nor shame, nor loss of maidenhead; Yet this enjoys before it woo, And pamper’d swells with one blood made of two; And this, alas! Is more than we would do.”Since Valentine’s Day is coming up a little bit of John Donne’s “The Flea” goes someway of recognising that fact! Of course chatting up women in the 17th century was a far different endeavour but you understand the audacity invoked by comparing how a flea sucking blood from two people should have any bearing on whether they should further their relationship. As we say back home; ‘God loves a trier, but he hates a chancer!’ In that mind actually, trying and chancing my way through data I was slightly stuck in a rut but now it appears I’ve gone from one rut to an even bigger one in terms of processing high resolution X-ray fluorescence (XRF) data from the ITRAX™ core scanner.
In order for the data to be of any use at all in interpreting environmental changes, sedimentary processes and indeed sedimentary provenance signals, the element intensities measured by XRF core scanners need to be converted to some form of element concentrations, essential for quantitative applications and this is where we see some problems emerging. For the most part the results from XRF core scanners are usually presented in the form of count rates (expressed as counts per unit time per unit area), or as ratios of counts, count rates, or intensities of elements (Richter et al., 2006; Rothwell et al., 2006; Thomson et al., 2006 cited in Welte and Tjallingii, 2008).
Regular calibration exercises tend to lead to the constant-sum constraint, which is a major headache whereby basic assumptions upon which standard statistical analyses are designed appear to be neglected leading to major issues down the road (Kucera and Malmgren, 1998). The constant-sum constraint (CSC) is a mathematical property found in any compositional dataset, this is a dataset in which all variables in a sample sum to a unit value (1 or 100%) (Kucera and Malmgren, 1998). The issues we have here is that individual variables are thus not allowed to vary independently, so in effect you would see for example that as the proportion of one component variable increases, the proportion of the remaining components must decrease consequently (Kucera and Malmgren, 1998). Having such forced correlations really isn’t cricket in any sense of the word, thus the attempts that have been made in most previous geochemical studies have often avoided this elephant in the room. Essentially most work previously done involved taking some form of ordinary least-squares linear regression whereby the correlation coefficients (those r-squared values) are ascribed to being either low, attributed to the inhomogeneity of the sample relative to instrument detection limits or being rather high (greater than 0.85) showing a good fit of the data (Welte and Tjallingii, 2008). The best way to imagine this problem is like comparing two rock specimens in order to determine whether or not they are different, in that if the two specimens do not have the same mass it would be silly to compare them in terms of the amount of chemical elements present (Welte and Tjallingii, 2008). Any sort of reasonable comparison needs closure and the best way to do that is through a ratio, so for example if both rock specimens are Mourne granites, the ratio of silica to aluminium should be identical. So with that key word ‘ratio’ in mind, that’s where I am currently situated regarding analysis of my XRF data. It’s thanks to John Aitchison that the foundations of a new approach to the statistical analysis of compositional data was developed in the early 1980s (Aitchison 1981, 1982, 1983, 1984), leading to his monograph that brought this work together (Aitchison 1986). The approach Aitchison developed was based on log-ratios, in which the relative magnitudes and variations of components, rather than their absolute values provide the key to analysing compositional data (Pawlowsky-Glahn and Egozcue, 2006).
So, while the dinner reservations are being made, Interflora are whizzing about Belfast, and Barry White is getting a belt out on the stereo over a glass or two of merlot, I’ll be cracking away at this aul calibration for the next while. “Oh but it’s all worth it in the end” as that voice in my head reminds me, albeit with a diminishing volume as time passes by inexorably!
Till next time folks!
Rory Flood, PhD Student, Queen’s University Belfast, School of Geography, Archaeology and Palaeoecology (GAP), http://www.qub.ac.uk/schools/gap/
We need to make clear, that at the time of writing, there are no ISO or EN standards which deal with the sampling of groundwater for PFAS.
Read MoreEnvironmental monitoring has developed considerably over the years. From the time when a consultant went out monthly or quarterly with a dip tape to monitor the groundwater level in a borehole, wind forward...
Read MoreThe interest in Nitrates is nothing new. One way or another we have been measuring them for half a century.
Read MoreA small selection of our environmental equipment